Import all necessary packages
# For Matrix and mathematical operations.
import numpy as np
# For visualizing graphs
import matplotlib
import matplotlib.pyplot as plt
%matplotlib inline
import seaborn as sns
from sklearn.metrics import classification_report, confusion_matrix
from sklearn import metrics
Data Preparation
X = np.array([
[0, 0],
[0, 1],
[1, 0],
[1, 1]])
y = np.array([0, 1, 2, 3])
def plot_multiclass_scatted_data(X, y):
plt.style.use('ggplot')
#plt.style.use(['dark_background'])
#plt.style.use('classic')
plot_colors = "rgby"
class_names = "0123"
cmap = plt.get_cmap('Paired')
fig = plt.figure(figsize=(6, 6))
x_min, x_max = X[:, 0].min() - 1, X[:, 0].max() + 1
y_min, y_max = X[:, 1].min() - 1, X[:, 1].max() + 1
# Plot the training points
for i, n, c in zip(range(4), class_names, plot_colors):
idx = np.where(y == i)
plt.scatter(X[idx, 0], X[idx, 1], c=c, cmap=cmap, label="Class %s" % n)
plt.xlim(x_min, x_max)
plt.ylim(y_min, y_max)
plt.legend(loc='upper right')
plt.xlabel('x1')
plt.ylabel('x2')
plt.title('Multiclass Data Scatter Plot')
plt.tight_layout()
plt.show()
plot_multiclass_scatted_data(X, y)

print("X shape ", X.shape)
print("y shape ", y.shape)
output:
X shape (4, 2) y shape (4,)
X_train = X.T
# Creating labels by one-hot encoding of y
digits = 4
examples = y.shape[0]
y_train = y.reshape(1, examples)
Y_train = np.eye(digits)[y_train.astype('int32')]
Y_train = Y_train.T.reshape(digits, examples)
print("X_train shape", X_train.shape)
print("Y_train shape", Y_train.shape)
print("X_train dataset\n ", X_train)
print("Y_train dataset\n", Y_train)
output:
X_train shape (2, 4)
Y_train shape (4, 4)
X_train dataset
[[0 0 1 1]
[0 1 0 1]]
Y_train dataset [[1. 0. 0. 0.] [0. 1. 0. 0.] [0. 0. 1. 0.] [0. 0. 0. 1.]]
Neural Network Model Setup Step-by-step analysis of neural network outputs
# Neural Network Model
# initialization
np.random.seed(48)
n_features = X_train.shape[0]
n_out = Y_train.shape[0]
params = { "W": np.zeros((n_out, n_features)),
"b": np.zeros((n_out, 1)) }
count=0
print("W shape", params["W"].shape)
print("b shape", params["b"].shape)
count+=1
learning_rate=1
cache = {}
cache["Z"] = np.dot(params["W"], X_train) + params["b"]
cache["P"] = softmax(cache["Z"])
cost = CrossEntropy(Y_train, cache["P"])
m_batch = X_train.shape[1]
dZ = (1./m_batch) * (cache["P"] - Y_train)
dW = np.dot(dZ, X_train.T)
db = np.sum(dZ, axis=1, keepdims=True)
print("Epoch", count)
print("X_train", X_train)
print("Z", cache["Z"])
print("P", cache["P"])
print("Cost {:.3f}".format(cost))
print("dZ", dZ)
print("dW", dW)
print("db", db)
print("W", params["W"])
print("b", params["b"])
params["W"] = params["W"] - learning_rate * dW
params["b"] = params["b"] - learning_rate * db
print("W*", params["W"])
print("b*", params["b"])
Neural Network Training
# Neural Network Model
# initialization
np.random.seed(48)
n_features = X_train.shape[0]
n_out = Y_train.shape[0]
params = { "W": np.zeros((n_out, n_features)),
"b": np.zeros((n_out, 1)) }
# Hyper-parameters
learning_rate = 1
number_of_epoch=60
m_batch = X_train.shape[1]
# initially empty list, this will store all the training costs
costs = []
# Start training
for epoch in range(number_of_epoch):
cache = feed_forward(X_train, params)
grads = back_propagate_softmax(X_train, Y_train, cache)
params = update_parameters(params, grads, learning_rate)
cost = CrossEntropy(Y_train, cache["P"])
if (epoch % 1) == 0 or epoch == number_of_epoch - 1:
print("Cost at epoch#{}: {:.3f}".format(epoch+1, cost))
costs.append(cost)
output:
Cost at epoch#1: 1.386
Cost at epoch#2: 1.269
Cost at epoch#3: 1.175
Cost at epoch#4: 1.094
Cost at epoch#5: 1.023
Cost at epoch#6: 0.959
Cost at epoch#7: 0.902
Cost at epoch#8: 0.850
Cost at epoch#9: 0.803
Cost at epoch#10: 0.760
Cost at epoch#11: 0.720
Cost at epoch#12: 0.684
Cost at epoch#13: 0.651
Cost at epoch#14: 0.620
Cost at epoch#15: 0.592
Cost at epoch#16: 0.566
Cost at epoch#17: 0.542
Cost at epoch#18: 0.520
Cost at epoch#19: 0.499
Cost at epoch#20: 0.479
Cost at epoch#21: 0.461
Cost at epoch#22: 0.444
Cost at epoch#23: 0.428
Cost at epoch#24: 0.413
...
...
Performance Evaluation
def plot_learning_curve(costs, learning_rate, total_epochs, save=False):
# plot the cost
plt.figure()
#plt.style.use("fivethirtyeight")
plt.style.use('seaborn-whitegrid')
# the steps at with costs were recorded
steps = int(total_epochs / len(costs))
plt.ylabel('Cost')
plt.xlabel('Iterations ')
plt.title("Learning rate =" + str(learning_rate))
plt.plot(np.squeeze(costs))
locs, labels = plt.xticks()
# change x labels of the plot
plt.xticks(locs[1:-1], tuple(np.array(locs[1:-1], dtype='int')*steps))
plt.xticks()
if save:
plt.savefig('Cost_Curve.png', bbox_inches='tight')
plt.show()
plot_learning_curve(costs, learning_rate,
number_of_epoch, save=True)
cache = feed_forward(X_train, params)
predictions = np.argmax(cache["P"], axis=0)
labels = np.argmax(Y_train, axis=0)
print("Input: \n", X_train)
print("Actual Output: \n ", Y_train)
print("Output Probabilities \n", cache["P"])
print("Labeled Output: ", labels)
print("Predicted Output (argmax):", predictions)
def make_confusion_matrix(cf,
group_names=None,
categories='auto',
count=True,
percent=False,
cbar=True,
xyticks=True,
xyplotlabels=True,
sum_stats=True,
figsize=None,
cmap='Blues',
title=None):
# CODE TO GENERATE TEXT INSIDE EACH SQUARE
blanks = ['' for i in range(cf.size)]
if group_names and len(group_names)==cf.size:
group_labels = ["{}\n".format(value) for value in group_names]
else:
group_labels = blanks
if count:
group_counts = ["{0:0.0f}\n".format(value) for value in cf.flatten()]
else:
group_counts = blanks
if percent:
group_percentages = ["{0:.2%}".format(value) for value in cf.flatten()/np.sum(cf)]
else:
group_percentages = blanks
box_labels = [f"{v1}{v2}{v3}".strip() for v1, v2, v3 in zip(group_labels,group_counts,group_percentages)]
box_labels = np.asarray(box_labels).reshape(cf.shape[0],cf.shape[1])
# CODE TO GENERATE SUMMARY STATISTICS & TEXT FOR SUMMARY STATS
if sum_stats:
#Accuracy is sum of diagonal divided by total observations
accuracy = np.trace(cf) / float(np.sum(cf))
#if it is a binary confusion matrix, show some more stats
if len(cf)==2:
#Metrics for Binary Confusion Matrices
precision = cf[1,1] / sum(cf[:,1])
recall = cf[1,1] / sum(cf[1,:])
f1_score = 2*precision*recall / (precision + recall)
stats_text = "\n\nAccuracy={:0.3f}\nPrecision={:0.3f}\nRecall={:0.3f}\nF1 Score={:0.3f}".format(
accuracy,precision,recall,f1_score)
else:
stats_text = "\n\nAccuracy={:0.3f}".format(accuracy)
else:
stats_text = ""
# SET FIGURE PARAMETERS ACCORDING TO OTHER ARGUMENTS
if figsize==None:
#Get default figure size if not set
figsize = plt.rcParams.get('figure.figsize')
if xyticks==False:
#Do not show categories if xyticks is False
categories=False
# MAKE THE HEATMAP VISUALIZATION
plt.figure(figsize=figsize)
sns.heatmap(cf,annot=box_labels,fmt="",cmap=cmap,cbar=cbar,xticklabels=categories,yticklabels=categories)
if xyplotlabels:
plt.ylabel('True label')
plt.xlabel('Predicted label' + stats_text)
else:
plt.xlabel(stats_text)
if title:
plt.title(title)
cache = feed_forward(X_train, params)
predictions = np.argmax(cache["P"], axis=0)
labels = np.argmax(Y_train, axis=0)
print(classification_report(predictions, labels))
cf_matrix =confusion_matrix(predictions, labels)
make_confusion_matrix(cf_matrix, figsize=(8,6), cbar=True)
count_misclassified = (predictions != labels).sum()
print('Misclassified samples: {}'.format(count_misclassified))
accuracy = metrics.accuracy_score(predictions, labels)
print('Accuracy: {:.2f}'.format(accuracy))
def predict_multiclass(params, data_in):
cache = feed_forward(data_in, params)
probas = cache["P"]
# np.argmax returns the class number of
# highest probability distribution of an example
predictions = np.argmax(cache["P"], axis=0)
return predictions
def plot_multiclass_decision_boundary(model, X, y):
plt.style.use('ggplot')
# Plot the decision boundaries
plot_colors = "rgby"
class_names = "0123"
cmap = plt.get_cmap('Paired')
fig = plt.figure(figsize=(6, 6))
x_min, x_max = X[:, 0].min() - 1, X[:, 0].max() + 1
y_min, y_max = X[:, 1].min() - 1, X[:, 1].max() + 1
x_span = np.linspace(x_min, x_max, 1000)
y_span = np.linspace(y_min, y_max, 1000)
xx, yy = np.meshgrid(x_span, y_span)
# Predict the function value for the whole grid
# np.c: Translates slice objects to concatenation along the second axis.
prediction_data = np.c_[xx.ravel(), yy.ravel()]
print("XX: {}, XX.ravel: {}".format (xx.shape, xx.ravel().shape))
print("yy: {}, yy.ravel: {}".format (yy.shape, yy.ravel().shape))
print("np.c concated shape{} as Predidction_data".format (prediction_data.shape))
print("Prediction Data: Before Transpose", prediction_data.shape)
prediction_data = prediction_data.T
print("Prediction Data: After Transpose", prediction_data.shape)
Z = model(prediction_data)
print("Z: Before Reshape", Z.shape)
Z = Z.reshape(xx.shape)
print("Z: After Reshape", Z.shape)
plt.contourf(xx, yy, Z, cmap=cmap, alpha=0.5)
plt.axis("tight")
# Plot the training points
print(y.shape[0])
for i, n, c in zip(range(y.shape[0]), class_names, plot_colors):
idx = np.where(y == i)
plt.scatter(X[idx, 0], X[idx, 1], c=c, cmap=cmap, label="Class %s" % n)
plt.xlim(x_min, x_max)
plt.ylim(y_min, y_max)
plt.legend(loc='upper right')
plt.xlabel('x1')
plt.ylabel('x2')
plt.title('Decision Boundary')
plt.tight_layout()
plt.show()
plot_multiclass_decision_boundary(lambda x: predict_multiclass(params, x), X, y)
Further Evaluation
from numpy.random import RandomState
plt.style.use('ggplot')
#plt.style.use(['dark_background'])
#plt.style.use('classic')
RNG = RandomState(42)
# Construct an example multiclass dataset
n_events = 1000
spread=1
class_0 = RNG.multivariate_normal(
[-2, -2], np.diag([spread, spread]), n_events)
class_1 = RNG.multivariate_normal(
[-2, 2], np.diag([spread, spread]), n_events)
class_2 = RNG.multivariate_normal(
[2, -2], np.diag([spread, spread]), n_events)
class_3 = RNG.multivariate_normal(
[2, 2], np.diag([spread, spread]), n_events)
X_test = np.concatenate([class_0, class_1, class_2, class_3])
y_test = np.ones(X_test.shape[0])
w = RNG.randint(1, 10, n_events * 4)
y_test[:1000]*= 0
y_test[2000:3000]*= 2
y_test[3000:4000]*= 3
permute = RNG.permutation(y_test.shape[0])
X_test = X_test[permute]
y_test = y_test[permute]
plot_multiclass_scatted_data(X_test, y_test)
output:
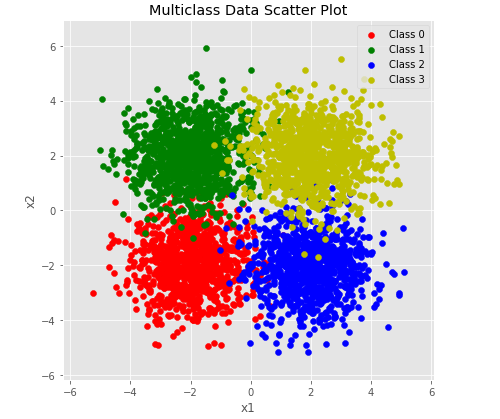
plot_multiclass_decision_boundary(lambda x: predict_multiclass(params, x), X_test, y_test)
output:
XX: (1000, 1000), XX.ravel: (1000000,)
yy: (1000, 1000), yy.ravel: (1000000,)
np.c concated shape(1000000, 2) as Predidction_data
Prediction Data: Before Transpose (1000000, 2)
Prediction Data: After Transpose (2, 1000000)
Z: Before Reshape (1000000,)
Z: After Reshape (1000, 1000)
4000

To get any other help you can contact us at:
or comment in below comment section and get instant help with an affordable price.
Comments