Intrusion Detection system using Multiple layer Perceptron(MLP) And Decision Tree(DT)
- realcode4you
- Aug 22, 2021
- 4 min read
Import All Related Libraries
#import Libraries
import numpy as np
import pandas as pd
import matplotlib.pyplot as plt
import seaborn as sns
from tensorflow.keras.utils import get_file
Read Data
#Read Data
try:
path = get_file('kddcup.data_10_percent.gz', origin=
'http://kdd.ics.uci.edu/databases/kddcup99/kddcup.data_10_percent.gz')
except:
print('Error downloading')
raise
print(path)
# This file is a CSV, just no CSV extension or headers
# Download from: http://kdd.ics.uci.edu/databases/kddcup99/kddcup99.html
df = pd.read_csv(path, header=None)
#Replace Null Value
print("Read {} rows.".format(len(df)))
# df = df.sample(frac=0.1, replace=False) # Uncomment this line to
# sample only 10% of the dataset
df.dropna(inplace=True,axis=1) # For now, just drop NA's
# (rows with missing values)
# The CSV file has no column heads, so add them
df.columns = [
'duration',
'protocol_type',
'service',
'flag',
'src_bytes',
'dst_bytes',
'land',
'wrong_fragment',
'urgent',
'hot',
'num_failed_logins',
'logged_in',
'num_compromised',
'root_shell',
'su_attempted',
'num_root',
'num_file_creations',
'num_shells',
'num_access_files',
'num_outbound_cmds',
'is_host_login',
'is_guest_login',
'count',
'srv_count',
'serror_rate',
'srv_serror_rate',
'rerror_rate',
'srv_rerror_rate',
'same_srv_rate',
'diff_srv_rate',
'srv_diff_host_rate',
'dst_host_count',
'dst_host_srv_count',
'dst_host_same_srv_rate',
'dst_host_diff_srv_rate',
'dst_host_same_src_port_rate',
'dst_host_srv_diff_host_rate',
'dst_host_serror_rate',
'dst_host_srv_serror_rate',
'dst_host_rerror_rate',
'dst_host_srv_rerror_rate',
'outcome'
]
pd.set_option('display.max_columns', 5)
pd.set_option('display.max_rows', 5)
# display 5 rows
display(df[0:5])
Output:
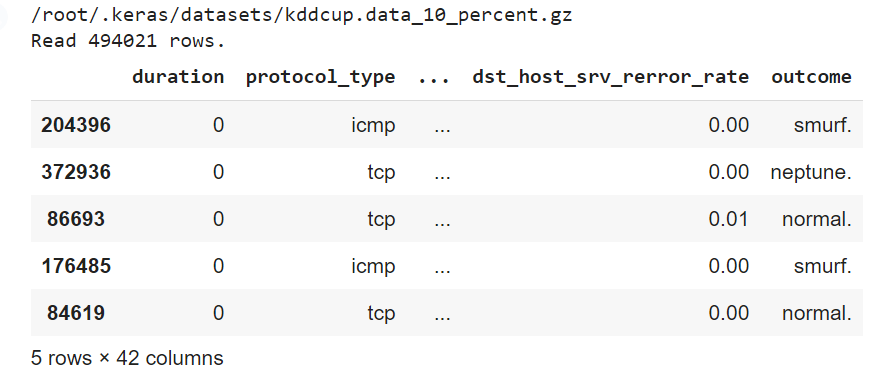
Check "Outcome" column data
df['outcome'].unique()
Output:
array(['smurf.', 'neptune.', 'normal.', 'nmap.', 'back.', 'ipsweep.', 'satan.', 'teardrop.', 'warezclient.', 'pod.', 'portsweep.', 'ftp_write.', 'guess_passwd.', 'warezmaster.', 'land.', 'loadmodule.', 'buffer_overflow.'], dtype=object)
import pandas as pd
import os
import numpy as np
from sklearn import metrics
from scipy.stats import zscore
def expand_categories(values):
result = []
s = values.value_counts()
t = float(len(values))
for v in s.index:
result.append("{}:{}%".format(v,round(100*(s[v]/t),2)))
return "[{}]".format(",".join(result))
def analyze(df):
print()
cols = df.columns.values
total = float(len(df))
print("{} rows".format(int(total)))
for col in cols:
uniques = df[col].unique()
unique_count = len(uniques)
if unique_count>100:
print("** {}:{} ({}%)".format(col,unique_count,int(((unique_count)/total)*100)))
else:
print("** {}:{}".format(col,expand_categories(df[col])))
expand_categories(df[col])
analyze(df)
Output:
494021 rows ** duration:2495 (0%) ** protocol_type:[icmp:57.41%,tcp:38.47%,udp:4.12%] ** service:[ecr_i:56.96%,private:22.45%,http:13.01%,smtp:1.97%,other:1.46%,domain_u:1.19%,ftp_data:0.96%,eco_i:0.33%,ftp:0.16%,finger:0.14%,urp_i:0.11%,telnet:0.1%,ntp_u:0.08%,auth:0.07%,pop_3:0.04%,time:0.03%,csnet_ns:0.03%,remote_job:0.02%,imap4:0.02%,gopher:0.02%,domain:0.02%,discard:0.02%,systat:0.02%,iso_tsap:0.02%,echo:0.02%,shell:0.02%,rje:0.02%,whois:0.02%,sql_net:0.02%,printer:0.02%,nntp:0.02%,courier:0.02%,netbios_ssn:0.02%,mtp:0.02%,sunrpc:0.02%,uucp:0.02%,uucp_path:0.02%,klogin:0.02%,bgp:0.02%,vmnet:0.02%,nnsp:0.02%,supdup:0.02%,ssh:0.02%,login:0.02%,hostnames:0.02%,daytime:0.02%,efs:0.02%,link:0.02%,netbios_ns:0.02%,pop_2:0.02%,ldap:0.02%,http_443:0.02%,netbios_dgm:0.02%,exec:0.02%,name:0.02%,kshell:0.02%,ctf:0.02%,netstat:0.02%,Z39_50:0.02%,IRC:0.01%,urh_i:0.0%,X11:0.0%,tim_i:0.0%,red_i:0.0%,tftp_u:0.0%,pm_dump:0.0%] ** flag:[SF:76.6%,S0:17.61%,REJ:5.44%,RSTR:0.18%,RSTO:0.12%,SH:0.02%,S1:0.01%,S2:0.0%,RSTOS0:0.0%,S3:0.0%,OTH:0.0%] ** src_bytes:3300 (0%) ** dst_bytes:10725 (2%) ** land:[0:100.0%,1:0.0%] ** wrong_fragment:[0:99.75%,3:0.2%,1:0.05%] ** urgent:[0:100.0%,1:0.0%,3:0.0%,2:0.0%] ** hot:[0:99.35%,2:0.44%,28:0.06%,1:0.05%,4:0.02%,6:0.02%,5:0.01%,3:0.01%,14:0.01%,30:0.01%,22:0.01%,19:0.0%,18:0.0%,24:0.0%,20:0.0%,7:0.0%,17:0.0%,12:0.0%,15:0.0%,16:0.0%,10:0.0%,9:0.0%] ** num_failed_logins:[0:99.99%,1:0.01%,2:0.0%,5:0.0%,4:0.0%,3:0.0%] ** logged_in:[0:85.18%,1:14.82%] ** num_compromised:[0:99.55%,1:0.44%,2:0.0%,4:0.0%,3:0.0%,6:0.0%,5:0.0%,7:0.0%,12:0.0%,9:0.0%,11:0.0%,767:0.0%,238:0.0%,16:0.0%,18:0.0%,275:0.0%,21:0.0%,22:0.0%,281:0.0%,38:0.0%,102:0.0%,884:0.0%,13:0.0%] ** root_shell:[0:99.99%,1:0.01%] ** su_attempted:[0:100.0%,2:0.0%,1:0.0%] ** num_root:[0:99.88%,1:0.05%,9:0.03%,6:0.03%,2:0.0%,5:0.0%,4:0.0%,3:0.0%,119:0.0%,7:0.0%,993:0.0%,268:0.0%,14:0.0%,16:0.0%,278:0.0%,39:0.0%,306:0.0%,54:0.0%,857:0.0%,12:0.0%] ** num_file_creations:[0:99.95%,1:0.04%,2:0.01%,4:0.0%,16:0.0%,9:0.0%,5:0.0%,7:0.0%,8:0.0%,28:0.0%,25:0.0%,12:0.0%,14:0.0%,15:0.0%,20:0.0%,21:0.0%,22:0.0%,10:0.0%] ** num_shells:[0:99.99%,1:0.01%,2:0.0%] ** num_access_files:[0:99.91%,1:0.09%,2:0.01%,3:0.0%,8:0.0%,6:0.0%,4:0.0%] ** num_outbound_cmds:[0:100.0%] ** is_host_login:[0:100.0%] ** is_guest_login:[0:99.86%,1:0.14%] ** count:490 (0%) ** srv_count:470 (0%) ** serror_rate:[0.0:81.94%,1.0:17.52%,0.99:0.06%,0.08:0.03%,0.05:0.03%,0.07:0.03%,0.06:0.03%,0.14:0.02%,0.04:0.02%,0.01:0.02%,0.09:0.02%,0.1:0.02%,0.03:0.02%,0.11:0.02%,0.13:0.02%,0.5:0.02%,0.12:0.02%,0.2:0.01%,0.25:0.01%,0.02:0.01%,0.17:0.01%,0.33:0.01%,0.15:0.01%,0.22:0.01%,0.18:0.01%,0.23:0.01%,0.16:0.01%,0.21:0.01%,0.19:0.0%,0.27:0.0%,0.98:0.0%,0.44:0.0%,0.29:0.0%,0.24:0.0%,0.97:0.0%,0.96:0.0%,0.31:0.0%,0.26:0.0%,0.67:0.0%,0.36:0.0%,0.65:0.0%,0.94:0.0%,0.28:0.0%,0.79:0.0%,0.95:0.0%,0.53:0.0%,0.81:0.0%,0.62:0.0%,0.85:0.0%,0.6:0.0%,0.64:0.0%,0.88:0.0%,0.68:0.0%,0.52:0.0%,0.66:0.0%,0.71:0.0%,0.93:0.0%,0.57:0.0%,0.63:0.0%,0.83:0.0%,0.78:0.0%,0.75:0.0%,0.51:0.0%,0.58:0.0%,0.56:0.0%,0.55:0.0%,0.3:0.0%,0.76:0.0%,0.86:0.0%,0.74:0.0%,0.35:0.0%,0.38:0.0%,0.54:0.0%,0.72:0.0%,0.84:0.0%,0.69:0.0%,0.61:0.0%,0.59:0.0%,0.42:0.0%,0.32:0.0%,0.82:0.0%,0.77:0.0%,0.7:0.0%,0.91:0.0%,0.92:0.0%,0.4:0.0%,0.73:0.0%,0.9:0.0%,0.34:0.0%,0.8:0.0%,0.89:0.0%,0.87:0.0%] ** srv_serror_rate:[0.0:82.12%,1.0:17.62%,0.03:0.03%,0.04:0.02%,0.05:0.02%,0.06:0.02%,0.02:0.02%,0.5:0.02%,0.08:0.01%,0.07:0.01%,0.25:0.01%,0.33:0.01%,0.17:0.01%,0.09:0.01%,0.1:0.01%,0.2:0.01%,0.11:0.01%,0.12:0.01%,0.14:0.01%,0.01:0.0%,0.67:0.0%,0.92:0.0%,0.18:0.0%,0.94:0.0%,0.95:0.0%,0.58:0.0%,0.88:0.0%,0.75:0.0%,0.19:0.0%,0.4:0.0%,0.76:0.0%,0.83:0.0%,0.91:0.0%,0.15:0.0%,0.22:0.0%,0.93:0.0%,0.85:0.0%,0.27:0.0%,0.86:0.0%,0.44:0.0%,0.35:0.0%,0.51:0.0%,0.36:0.0%,0.38:0.0%,0.21:0.0%,0.8:0.0%,0.9:0.0%,0.45:0.0%,0.16:0.0%,0.37:0.0%,0.23:0.0%] ** rerror_rate:[0.0:94.12%,1.0:5.46%,0.86:0.02%,0.87:0.02%,0.92:0.02%,0.25:0.02%,0.95:0.02%,0.9:0.02%,0.5:0.02%,0.91:0.02%,0.88:0.01%,0.96:0.01%,0.33:0.01%,0.2:0.01%,0.93:0.01%,0.94:0.01%,0.01:0.01%,0.89:0.01%,0.85:0.01%,0.99:0.01%,0.82:0.01%,0.77:0.01%,0.17:0.01%,0.97:0.01%,0.02:0.01%,0.98:0.01%,0.03:0.01%,0.8:0.01%,0.78:0.01%,0.76:0.01%,0.75:0.0%,0.79:0.0%,0.84:0.0%,0.14:0.0%,0.05:0.0%,0.73:0.0%,0.81:0.0%,0.06:0.0%,0.71:0.0%,0.83:0.0%,0.67:0.0%,0.56:0.0%,0.08:0.0%,0.04:0.0%,0.1:0.0%,0.09:0.0%,0.12:0.0%,0.07:0.0%,0.11:0.0%,0.69:0.0%,0.74:0.0%,0.64:0.0%,0.4:0.0%,0.72:0.0%,0.7:0.0%,0.6:0.0%,0.29:0.0%,0.22:0.0%,0.62:0.0%,0.65:0.0%,0.21:0.0%,0.68:0.0%,0.37:0.0%,0.19:0.0%,0.43:0.0%,0.58:0.0%,0.35:0.0%,0.24:0.0%,0.31:0.0%,0.23:0.0%,0.27:0.0%,0.28:0.0%,0.26:0.0%,0.36:0.0%,0.34:0.0%,0.66:0.0%,0.32:0.0%] ** srv_rerror_rate:[0.0:93.99%,1.0:5.69%,0.33:0.05%,0.5:0.04%,0.25:0.04%,0.2:0.03%,0.17:0.03%,0.14:0.01%,0.04:0.01%,0.03:0.01%,0.12:0.01%,0.02:0.01%,0.06:0.01%,0.05:0.01%,0.07:0.01%,0.4:0.01%,0.67:0.01%,0.08:0.01%,0.11:0.01%,0.29:0.01%,0.09:0.0%,0.1:0.0%,0.75:0.0%,0.6:0.0%,0.01:0.0%,0.22:0.0%,0.71:0.0%,0.86:0.0%,0.83:0.0%,0.73:0.0%,0.81:0.0%,0.88:0.0%,0.96:0.0%,0.92:0.0%,0.18:0.0%,0.43:0.0%,0.79:0.0%,0.93:0.0%,0.13:0.0%,0.27:0.0%,0.38:0.0%,0.94:0.0%,0.95:0.0%,0.37:0.0%,0.85:0.0%,0.8:0.0%,0.62:0.0%,0.82:0.0%,0.69:0.0%,0.21:0.0%,0.87:0.0%] ** same_srv_rate:[1.0:77.34%,0.06:2.27%,0.05:2.14%,0.04:2.06%,0.07:2.03%,0.03:1.93%,0.02:1.9%,0.01:1.77%,0.08:1.48%,0.09:1.01%,0.1:0.8%,0.0:0.73%,0.12:0.73%,0.11:0.67%,0.13:0.66%,0.14:0.51%,0.15:0.35%,0.5:0.29%,0.16:0.25%,0.17:0.17%,0.33:0.12%,0.18:0.1%,0.2:0.08%,0.19:0.07%,0.67:0.05%,0.25:0.04%,0.21:0.04%,0.99:0.03%,0.22:0.03%,0.24:0.02%,0.23:0.02%,0.4:0.02%,0.98:0.02%,0.75:0.02%,0.27:0.02%,0.26:0.01%,0.8:0.01%,0.29:0.01%,0.38:0.01%,0.86:0.01%,0.3:0.01%,0.31:0.01%,0.44:0.01%,0.83:0.01%,0.36:0.01%,0.28:0.01%,0.43:0.01%,0.6:0.01%,0.42:0.01%,0.97:0.01%,0.32:0.01%,0.35:0.01%,0.45:0.01%,0.47:0.01%,0.88:0.0%,0.48:0.0%,0.39:0.0%,0.52:0.0%,0.46:0.0%,0.37:0.0%,0.41:0.0%,0.89:0.0%,0.34:0.0%,0.92:0.0%,0.54:0.0%,0.53:0.0%,0.94:0.0%,0.95:0.0%,0.57:0.0%,0.96:0.0%,0.64:0.0%,0.71:0.0%,0.56:0.0%,0.62:0.0%,0.78:0.0%,0.9:0.0%,0.49:0.0%,0.91:0.0%,0.55:0.0%,0.65:0.0%,0.73:0.0%,0.58:0.0%,0.59:0.0%,0.93:0.0%,0.76:0.0%,0.51:0.0%,0.77:0.0%,0.82:0.0%,0.81:0.0%,0.74:0.0%,0.69:0.0%,0.79:0.0%,0.72:0.0%,0.7:0.0%,0.85:0.0%,0.68:0.0%,0.61:0.0%,0.63:0.0%,0.87:0.0%] ** diff_srv_rate:[0.0:77.33%,0.06:10.69%,0.07:5.83%,0.05:3.89%,0.08:0.66%,1.0:0.48%,0.04:0.19%,0.67:0.13%,0.5:0.12%,0.09:0.08%,0.6:0.06%,0.12:0.05%,0.1:0.04%,0.11:0.04%,0.14:0.03%,0.4:0.02%,0.13:0.02%,0.29:0.02%,0.01:0.02%,0.15:0.02%,0.03:0.02%,0.33:0.02%,0.17:0.02%,0.25:0.02%,0.75:0.01%,0.2:0.01%,0.18:0.01%,0.16:0.01%,0.19:0.01%,0.02:0.01%,0.22:0.01%,0.21:0.01%,0.27:0.01%,0.96:0.01%,0.31:0.01%,0.38:0.01%,0.24:0.01%,0.23:0.01%,0.43:0.0%,0.52:0.0%,0.95:0.0%,0.44:0.0%,0.53:0.0%,0.36:0.0%,0.8:0.0%,0.57:0.0%,0.42:0.0%,0.3:0.0%,0.26:0.0%,0.28:0.0%,0.56:0.0%,0.99:0.0%,0.54:0.0%,0.62:0.0%,0.37:0.0%,0.55:0.0%,0.35:0.0%,0.41:0.0%,0.47:0.0%,0.89:0.0%,0.32:0.0%,0.71:0.0%,0.58:0.0%,0.46:0.0%,0.39:0.0%,0.51:0.0%,0.45:0.0%,0.97:0.0%,0.83:0.0%,0.7:0.0%,0.69:0.0%,0.78:0.0%,0.74:0.0%,0.64:0.0%,0.73:0.0%,0.82:0.0%,0.88:0.0%,0.86:0.0%] ** srv_diff_host_rate:[0.0:92.99%,1.0:1.64%,0.12:0.31%,0.5:0.29%,0.67:0.29%,0.33:0.25%,0.11:0.24%,0.25:0.23%,0.1:0.22%,0.14:0.21%,0.17:0.21%,0.08:0.2%,0.15:0.2%,0.18:0.19%,0.2:0.19%,0.09:0.19%,0.4:0.19%,0.07:0.17%,0.29:0.17%,0.13:0.16%,0.22:0.16%,0.06:0.14%,0.02:0.1%,0.05:0.1%,0.01:0.08%,0.21:0.08%,0.19:0.08%,0.16:0.07%,0.75:0.07%,0.27:0.06%,0.04:0.06%,0.6:0.06%,0.3:0.06%,0.38:0.05%,0.43:0.05%,0.23:0.05%,0.03:0.03%,0.24:0.02%,0.36:0.02%,0.31:0.02%,0.8:0.02%,0.57:0.01%,0.44:0.01%,0.28:0.01%,0.26:0.01%,0.42:0.0%,0.45:0.0%,0.62:0.0%,0.83:0.0%,0.71:0.0%,0.56:0.0%,0.35:0.0%,0.32:0.0%,0.37:0.0%,0.41:0.0%,0.47:0.0%,0.86:0.0%,0.55:0.0%,0.54:0.0%,0.88:0.0%,0.64:0.0%,0.46:0.0%,0.7:0.0%,0.77:0.0%] ** dst_host_count:256 (0%) ** dst_host_srv_count:256 (0%) ** dst_host_same_srv_rate:101 (0%) ** dst_host_diff_srv_rate:101 (0%) ** dst_host_same_src_port_rate:101 (0%) ** dst_host_srv_diff_host_rate:[0.0:89.45%,0.02:2.38%,0.01:2.13%,0.04:1.35%,0.03:1.34%,0.05:0.94%,0.06:0.39%,0.07:0.31%,0.5:0.15%,0.08:0.14%,0.09:0.13%,0.15:0.09%,0.11:0.09%,0.16:0.08%,0.13:0.08%,0.1:0.08%,0.14:0.07%,1.0:0.07%,0.17:0.07%,0.2:0.07%,0.12:0.07%,0.18:0.07%,0.25:0.05%,0.22:0.05%,0.19:0.05%,0.21:0.05%,0.24:0.03%,0.23:0.02%,0.26:0.02%,0.27:0.02%,0.33:0.02%,0.29:0.02%,0.51:0.02%,0.4:0.01%,0.28:0.01%,0.3:0.01%,0.67:0.01%,0.52:0.01%,0.31:0.01%,0.32:0.01%,0.38:0.01%,0.53:0.0%,0.43:0.0%,0.44:0.0%,0.34:0.0%,0.6:0.0%,0.36:0.0%,0.57:0.0%,0.35:0.0%,0.54:0.0%,0.37:0.0%,0.56:0.0%,0.55:0.0%,0.42:0.0%,0.46:0.0%,0.45:0.0%,0.41:0.0%,0.48:0.0%,0.39:0.0%,0.8:0.0%,0.7:0.0%,0.47:0.0%,0.62:0.0%,0.75:0.0%,0.58:0.0%] ** dst_host_serror_rate:[0.0:80.93%,1.0:17.56%,0.01:0.74%,0.02:0.2%,0.03:0.09%,0.09:0.05%,0.04:0.04%,0.05:0.04%,0.07:0.03%,0.08:0.03%,0.06:0.02%,0.14:0.02%,0.15:0.02%,0.11:0.02%,0.13:0.02%,0.16:0.02%,0.1:0.02%,0.12:0.01%,0.18:0.01%,0.25:0.01%,0.2:0.01%,0.17:0.01%,0.33:0.01%,0.99:0.01%,0.19:0.01%,0.31:0.01%,0.27:0.01%,0.5:0.0%,0.22:0.0%,0.98:0.0%,0.35:0.0%,0.28:0.0%,0.53:0.0%,0.24:0.0%,0.96:0.0%,0.3:0.0%,0.26:0.0%,0.97:0.0%,0.29:0.0%,0.94:0.0%,0.42:0.0%,0.32:0.0%,0.56:0.0%,0.55:0.0%,0.95:0.0%,0.6:0.0%,0.23:0.0%,0.93:0.0%,0.34:0.0%,0.85:0.0%,0.89:0.0%,0.21:0.0%,0.92:0.0%,0.58:0.0%,0.43:0.0%,0.9:0.0%,0.57:0.0%,0.91:0.0%,0.49:0.0%,0.82:0.0%,0.36:0.0%,0.87:0.0%,0.45:0.0%,0.62:0.0%,0.65:0.0%,0.46:0.0%,0.38:0.0%,0.61:0.0%,0.47:0.0%,0.76:0.0%,0.81:0.0%,0.54:0.0%,0.64:0.0%,0.44:0.0%,0.48:0.0%,0.72:0.0%,0.39:0.0%,0.52:0.0%,0.51:0.0%,0.67:0.0%,0.84:0.0%,0.73:0.0%,0.4:0.0%,0.69:0.0%,0.79:0.0%,0.41:0.0%,0.68:0.0%,0.88:0.0%,0.77:0.0%,0.75:0.0%,0.7:0.0%,0.8:0.0%,0.59:0.0%,0.71:0.0%,0.37:0.0%,0.86:0.0%,0.66:0.0%,0.78:0.0%,0.74:0.0%,0.83:0.0%] ** dst_host_srv_serror_rate:[0.0:81.16%,1.0:17.61%,0.01:0.99%,0.02:0.14%,0.03:0.03%,0.04:0.02%,0.05:0.01%,0.06:0.01%,0.08:0.0%,0.5:0.0%,0.07:0.0%,0.1:0.0%,0.09:0.0%,0.11:0.0%,0.17:0.0%,0.14:0.0%,0.12:0.0%,0.96:0.0%,0.33:0.0%,0.67:0.0%,0.97:0.0%,0.25:0.0%,0.98:0.0%,0.4:0.0%,0.75:0.0%,0.48:0.0%,0.83:0.0%,0.16:0.0%,0.93:0.0%,0.69:0.0%,0.2:0.0%,0.91:0.0%,0.78:0.0%,0.95:0.0%,0.8:0.0%,0.92:0.0%,0.68:0.0%,0.29:0.0%,0.38:0.0%,0.88:0.0%,0.3:0.0%,0.32:0.0%,0.94:0.0%,0.57:0.0%,0.63:0.0%,0.62:0.0%,0.31:0.0%,0.85:0.0%,0.56:0.0%,0.81:0.0%,0.74:0.0%,0.86:0.0%,0.13:0.0%,0.23:0.0%,0.18:0.0%,0.64:0.0%,0.46:0.0%,0.52:0.0%,0.66:0.0%,0.6:0.0%,0.84:0.0%,0.55:0.0%,0.9:0.0%,0.15:0.0%,0.79:0.0%,0.82:0.0%,0.87:0.0%,0.47:0.0%,0.53:0.0%,0.45:0.0%,0.42:0.0%,0.24:0.0%] ** dst_host_rerror_rate:101 (0%) ** dst_host_srv_rerror_rate:101 (0%) ** outcome:[smurf.:56.84%,neptune.:21.7%,normal.:19.69%,back.:0.45%,satan.:0.32%,ipsweep.:0.25%,portsweep.:0.21%,warezclient.:0.21%,teardrop.:0.2%,pod.:0.05%,nmap.:0.05%,guess_passwd.:0.01%,buffer_overflow.:0.01%,land.:0.0%,warezmaster.:0.0%,imap.:0.0%,rootkit.:0.0%,loadmodule.:0.0%,ftp_write.:0.0%,multihop.:0.0%,phf.:0.0%,perl.:0.0%,spy.:0.0%]
Decode Non-Numeric Data
def encode_numeric_zscore(df, name, mean=None, sd=None):
if mean is None:
mean = df[name].mean()
if sd is None:
sd = df[name].std()
df[name] = (df[name] - mean) / sd
# Encode text values to dummy variables(i.e. [1,0,0],
# [0,1,0],[0,0,1] for red,green,blue)
def encode_text_dummy(df, name):
dummies = pd.get_dummies(df[name])
for x in dummies.columns:
dummy_name = f"{name}-{x}"
df[dummy_name] = dummies[x]
df.drop(name, axis=1, inplace=True)
# Now encode the feature vector
encode_numeric_zscore(df, 'duration')
encode_text_dummy(df, 'protocol_type')
encode_text_dummy(df, 'service')
encode_text_dummy(df, 'flag')
encode_numeric_zscore(df, 'src_bytes')
encode_numeric_zscore(df, 'dst_bytes')
encode_text_dummy(df, 'land')
encode_numeric_zscore(df, 'wrong_fragment')
encode_numeric_zscore(df, 'urgent')
encode_numeric_zscore(df, 'hot')
encode_numeric_zscore(df, 'num_failed_logins')
encode_text_dummy(df, 'logged_in')
encode_numeric_zscore(df, 'num_compromised')
encode_numeric_zscore(df, 'root_shell')
encode_numeric_zscore(df, 'su_attempted')
encode_numeric_zscore(df, 'num_root')
encode_numeric_zscore(df, 'num_file_creations')
encode_numeric_zscore(df, 'num_shells')
encode_numeric_zscore(df, 'num_access_files')
encode_numeric_zscore(df, 'num_outbound_cmds')
encode_text_dummy(df, 'is_host_login')
encode_text_dummy(df, 'is_guest_login')
encode_numeric_zscore(df, 'count')
encode_numeric_zscore(df, 'srv_count')
encode_numeric_zscore(df, 'serror_rate')
encode_numeric_zscore(df, 'srv_serror_rate')
encode_numeric_zscore(df, 'rerror_rate')
encode_numeric_zscore(df, 'srv_rerror_rate')
encode_numeric_zscore(df, 'same_srv_rate')
encode_numeric_zscore(df, 'diff_srv_rate')
encode_numeric_zscore(df, 'srv_diff_host_rate')
encode_numeric_zscore(df, 'dst_host_count')
encode_numeric_zscore(df, 'dst_host_srv_count')
encode_numeric_zscore(df, 'dst_host_same_srv_rate')
encode_numeric_zscore(df, 'dst_host_diff_srv_rate')
encode_numeric_zscore(df, 'dst_host_same_src_port_rate')
encode_numeric_zscore(df, 'dst_host_srv_diff_host_rate')
encode_numeric_zscore(df, 'dst_host_serror_rate')
encode_numeric_zscore(df, 'dst_host_srv_serror_rate')
encode_numeric_zscore(df, 'dst_host_rerror_rate')
encode_numeric_zscore(df, 'dst_host_srv_rerror_rate')
# display 5 rows
df.dropna(inplace=True,axis=1)
df[0:5]
# This is the numeric feature vector, as it goes to the neural net
# Convert to numpy - Classification
x_columns = df.columns.drop('outcome')
x = df[x_columns].values
dummies = pd.get_dummies(df['outcome']) # Classification
outcomes = dummies.columns
num_classes = len(outcomes)
y = dummies.values
df.groupby('outcome')['outcome'].count()
Output:

Split Dataset and Fit It Into The Model
import pandas as pd
import io
import requests
import numpy as np
import os
from sklearn.model_selection import train_test_split
from sklearn import metrics
from tensorflow.keras.models import Sequential
from tensorflow.keras.layers import Dense, Activation
from tensorflow.keras.callbacks import EarlyStopping
# Create a test/train split. 25% test
# Split into train/test
x_train, x_test, y_train, y_test = train_test_split(
x, y, test_size=0.25, random_state=42)
# Create neural net
model = Sequential()
model.add(Dense(10, input_dim=x.shape[1], activation='relu'))
model.add(Dense(50, input_dim=x.shape[1], activation='relu'))
model.add(Dense(10, input_dim=x.shape[1], activation='relu'))
model.add(Dense(1, kernel_initializer='normal'))
model.add(Dense(y.shape[1],activation='softmax'))
model.compile(loss='categorical_crossentropy', optimizer='adam')
monitor = EarlyStopping(monitor='val_loss', min_delta=1e-3,
patience=5, verbose=1, mode='auto',
restore_best_weights=True)
model.fit(x_train,y_train,validation_data=(x_test,y_test),
callbacks=[monitor],verbose=2,epochs=2000)
Output:
Epoch 1/2000 11579/11579 - 20s - loss: 0.1157 - val_loss: 0.0389 Epoch 2/2000 11579/11579 - 19s - loss: 0.0349 - val_loss: 0.0296 Epoch 3/2000 11579/11579 - 18s - loss: 0.0298 - val_loss: 0.0307 Epoch 4/2000 11579/11579 - 19s - loss: 0.0276 - val_loss: 0.0266 Epoch 5/2000 11579/11579 - 19s - loss: 0.0284 - val_loss: 0.0230 Epoch 6/2000 11579/11579 - 17s - loss: 0.0244 - val_loss: 0.0239 Epoch 7/2000 11579/11579 - 17s - loss: 0.0258 - val_loss: 0.0228 Epoch 8/2000 11579/11579 - 17s - loss: 0.0231 - val_loss: 0.0211 Epoch 9/2000 11579/11579 - 19s - loss: 0.0243 - val_loss: 0.0215 Epoch 10/2000 11579/11579 - 17s - loss: 0.0208 - val_loss: 0.0293 Epoch 11/2000 11579/11579 - 17s - loss: 0.0267 - val_loss: 0.0201 Epoch 12/2000 11579/11579 - 17s - loss: 0.0213 - val_loss: 0.0230 Epoch 13/2000 11579/11579 - 20s - loss: 0.0224 - val_loss: 0.0198 Epoch 14/2000 11579/11579 - 17s - loss: 0.0199 - val_loss: 0.0221 Epoch 15/2000 11579/11579 - 19s - loss: 0.0213 - val_loss: 0.0190 Epoch 16/2000 11579/11579 - 19s - loss: 0.0196 - val_loss: 0.0264 Epoch 17/2000 11579/11579 - 17s - loss: 0.0214 - val_loss: 0.0210 Epoch 18/2000 11579/11579 - 19s - loss: 0.0194 - val_loss: 0.0190 Restoring model weights from the end of the best epoch. Epoch 00018: early stopping
<keras.callbacks.History at 0x7fe2f7957290>
Predict The Validation Score
pred = model.predict(x_test)
pred = np.argmax(pred,axis=1)
y_eval = np.argmax(y_test,axis=1)
score = metrics.accuracy_score(y_eval, pred)
print("Validation score: {}".format(score))
Output:
Validation score: 0.995473904101825
Decision Tree
from sklearn.model_selection import train_test_split
#x_train,x_test,y_train, y_test = train_test_split(random_state=0,test_size =0.2)
x_train, x_test, y_train, y_test = train_test_split(
x, y, test_size=0.25, random_state=42)
Fit Into The Model
from sklearn.tree import DecisionTreeClassifier
tr= DecisionTreeClassifier(max_depth=5)
tr = tr.fit(x_train,y_train)
Plot The Tree
from sklearn import tree
tree.plot_tree(tr)
Output:
[Text(243.8844827586207, 199.32, 'X[15] <= 0.134\ngini = 0.051\nsamples = 370515\nvalue = [[368853, 1662]\n[370496, 19]\n[370508, 7]\n[370472, 43]
...
...

y_pred=tr.predict(x_test)
print("Prediction of test data:",y_pred)
Ouput:
Prediction of test data:
[[0 0 0 ... 0 0 0]
[0 0 0 ... 0 0 0]
[0 0 0 ... 0 0 0]
...
[0 0 0 ... 0 0 0]
[0 0 0 ... 0 0 0]
[0 0 0 ... 0 0 0]]
y_test
Output:
array([[0, 0, 0, ..., 0, 0, 0],
[0, 0, 0, ..., 0, 0, 0],
[0, 0, 0, ..., 0, 0, 0],
...,
[0, 0, 0, ..., 0, 0, 0],
[0, 0, 0, ..., 0, 0, 0],
[0, 0, 0, ..., 0, 0, 0]], dtype=uint8)
from sklearn import metrics
print(metrics.accuracy_score(y_test,y_pred))
Output:
0.9908425501595064
Comments