Keras comes bundled with the Fashion-MNIST database of fashion articles which, like the MNIST digits dataset, provides 28-by-28 grayscale images. Fashion-MNIST contains clothing-article images labeled in 10 categories—0 (T-shirt/top), 1 (Trouser), 2 (Pullover), 3 (Dress), 4 (Coat), 5 (Sandal), 6 (Shirt), 7 (Sneaker), 8 (Bag), 9 (Ankle boot)—with 60,000 training samples and 10,000 testing samples. Modify the convnet example to load and process Fashion-MNIST rather than MNIST—this requires simply importing the correct module, loading the data then running the model with these images and labels, then re-run the entire example. How well does the model perform on Fashion-MNIST compared to MNIST? How do the training times compare?
Import Libraries
# baseline cnn model for fashion mnist
from numpy import mean
from numpy import std
from matplotlib import pyplot
from sklearn.model_selection import KFold
from keras.datasets import fashion_mnist
from tensorflow.keras.utils import to_categorical
from keras.models import Sequential
from keras.layers import Conv2D
from keras.layers import MaxPooling2D
from keras.layers import Dense
from keras.layers import Flatten
from tensorflow.keras.optimizers import SGD
Load Dataset
# load train and test dataset
def load_dataset():
# load dataset
(trainX, trainY), (testX, testY) = fashion_mnist.load_data()
# reshape dataset to have a single channel
trainX = trainX.reshape((trainX.shape[0], 28, 28, 1))
testX = testX.reshape((testX.shape[0], 28, 28, 1))
# one hot encode target values
trainY = to_categorical(trainY)
testY = to_categorical(testY)
return trainX, trainY, testX, testY
Scale pixels or Resizing
# scale pixels
def prep_pixels(train, test):
# convert from integers to floats
train_norm = train.astype('float32')
test_norm = test.astype('float32')
# normalize to range 0-1
train_norm = train_norm / 255.0
test_norm = test_norm / 255.0
# return normalized images
return train_norm, test_norm
Defining Model
# define cnn model
def define_model():
model = Sequential()
model.add(Conv2D(32, (3, 3), activation='relu', kernel_initializer='he_uniform', input_shape=(28, 28, 1)))
model.add(MaxPooling2D((2, 2)))
model.add(Flatten())
model.add(Dense(100, activation='relu', kernel_initializer='he_uniform'))
model.add(Dense(10, activation='softmax'))
# compile model
opt = SGD(lr=0.01, momentum=0.9)
model.compile(optimizer=opt, loss='categorical_crossentropy', metrics=['accuracy'])
return model
Evaluating Model Using K-fold Cross Validation
# evaluate a model using k-fold cross-validation
def evaluate_model(dataX, dataY, n_folds=5):
scores, histories = list(), list()
# prepare cross validation
kfold = KFold(n_folds, shuffle=True, random_state=1)
# enumerate splits
for train_ix, test_ix in kfold.split(dataX):
# define model
model = define_model()
# select rows for train and test
trainX, trainY, testX, testY = dataX[train_ix], dataY[train_ix], dataX[test_ix], dataY[test_ix]
# fit model
history = model.fit(trainX, trainY, epochs=5, batch_size=32, validation_data=(testX, testY), verbose=0)
# evaluate model
_, acc = model.evaluate(testX, testY, verbose=0)
print('> %.3f' % (acc * 100.0))
# append scores
scores.append(acc)
histories.append(history)
return scores, histories
Plot the Learning Curve
# plot diagnostic learning curves
def summarize_diagnostics(histories):
for i in range(len(histories)):
# plot accuracy
pyplot.subplot(212)
pyplot.title('Classification Accuracy')
pyplot.plot(histories[i].history['accuracy'], color='blue', label='train')
pyplot.plot(histories[i].history['val_accuracy'], color='orange', label='test')
pyplot.show()
Summarizing the Model Performance
# summarize model performance
def summarize_performance(scores):
# print summary
print('Accuracy: mean=%.3f std=%.3f, n=%d' % (mean(scores)*100, std(scores)*100, len(scores)))
# box and whisker plots of results
pyplot.show()
# run the test harness for evaluating a model
def run_test_harness():
# load dataset
trainX, trainY, testX, testY = load_dataset()
# prepare pixel data
trainX, testX = prep_pixels(trainX, testX)
# evaluate model
scores, histories = evaluate_model(trainX, trainY)
# learning curves
summarize_diagnostics(histories)
# summarize estimated performance
summarize_performance(scores)
# run the test harness for evaluating a model
def test_harness():
# load dataset
trainX, trainY, testX, testY = load_dataset()
# prepare pixel data
trainX, testX = prep_pixels(trainX, testX)
# evaluate model
scores, histories = evaluate_model(trainX, trainY)
# learning curves
summarize_diagnostics(histories)
# summarize estimated performance
summarize_performance(scores)
# entry point, run the test harness
test_harness()
Output:
Downloading data from https://storage.googleapis.com/tensorflow/tf-keras-datasets/train-labels-idx1-ubyte.gz 32768/29515 [=================================] - 0s 0us/step 40960/29515 [=========================================] - 0s 0us/step Downloading data from https://storage.googleapis.com/tensorflow/tf-keras-datasets/train-images-idx3-ubyte.gz 26427392/26421880 [==============================] - 0s 0us/step 26435584/26421880 [==============================] - 0s 0us/step Downloading data from https://storage.googleapis.com/tensorflow/tf-keras-datasets/t10k-labels-idx1-ubyte.gz 16384/5148 [===============================================================================================] - 0s 0us/step Downloading data from https://storage.googleapis.com/tensorflow/tf-keras-datasets/t10k-images-idx3-ubyte.gz 4423680/4422102 [==============================] - 0s 0us/step 4431872/4422102 [==============================] - 0s 0us/step
/usr/local/lib/python3.7/dist-packages/keras/optimizer_v2/optimizer_v2.py:356: UserWarning: The `lr` argument is deprecated, use `learning_rate` instead. "The `lr` argument is deprecated, use `learning_rate` instead.")
> 90.925
> 90.608
> 90.383
> 90.117
> 90.400
/usr/local/lib/python3.7/dist-packages/ipykernel_launcher.py:78: MatplotlibDeprecationWarning: Adding an axes using the same arguments as a previous axes currently reuses the earlier instance. In a future version, a new instance will always be created and returned. Meanwhile, this warning can be suppressed, and the future behavior ensured, by passing a unique label to each axes instance.
Output:
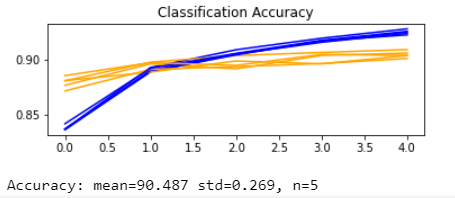
If you have any query related to this then comment in below comment section and if your need any other machine learning related help then send your requirement details at realcode4you@gmail.com and get instant help with an affordable price.
Comments