Here we have implement two Data Mining Techniques, below the some basic steps which used to implement Boston House Pricing data.
Data Mining Basics
1) Data pre-processing: Data categorical feature transformation, Data numerical feature normalization, Missing values imputation, and Cross-Validation.
Techniques
1) Decision Tree Regression
2) Support Vector Methodology
3) K-NN prediction modelling
4) K-mean clustering
5) Naïve Bayesian
Import Libraries
#import libraires
import pandas as pd
from sklearn.model_selection import train_test_split
from sklearn.cluster import KMeans
from sklearn.metrics import mean_absolute_error, make_scorer
import sklearn.metrics as metrics
import matplotlib.pyplot as plt
from sklearn.metrics import confusion_matrix
import seaborn as sns
import itertools
import numpy as np
import matplotlib.pyplot as plt
Read CSV
#Read Dataset
df = pd.read_csv("Boston Real Est.csv")
df
Output:
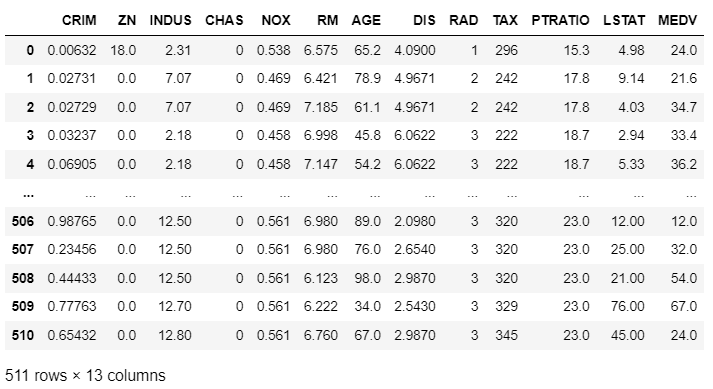
Checking Missing Values
#Checking Null Value
#Visualize for check null value
check_null_value = df.isnull()
sns.heatmap(check_null_value,yticklabels=False,cbar=False,cmap='viridis')
Output:
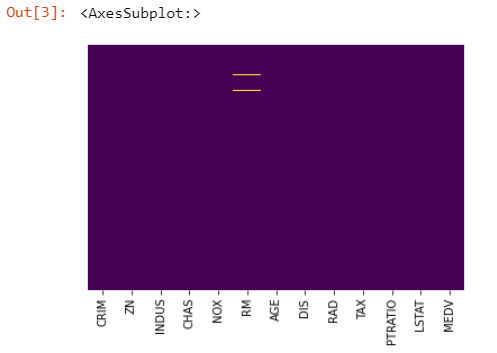
Show Dataset Columns:
#show data frame all columns
df.columns
Output:
Index(['CRIM', 'ZN', 'INDUS', 'CHAS', 'NOX', 'RM', 'AGE', 'DIS', 'RAD', 'TAX', 'PTRATIO', 'LSTAT', 'MEDV'], dtype='object')
In above heatmap we an see their is two missing values in RM Now we need to remove it using median
Remove Missing Values by median
#Remove Missing Values by Median
df['RM'].fillna(df['RM'].median(), inplace=True)
Checking Again Missing Values
#Now checking again Nan value
#Visualize for check null value
check_null_value = df.isnull()
sns.heatmap(check_null_value,yticklabels=False,cbar=False,cmap='viridis')
Output:
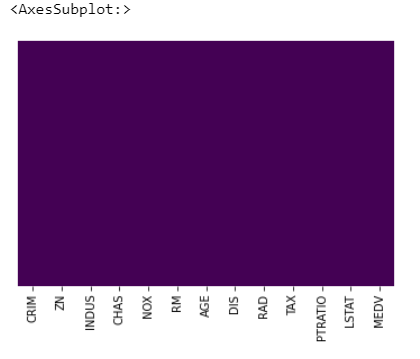
In above heat map we can she there are no missing values, all is replace by median.
#Checking shape of dataset
df.shape
Output:
(511, 13)
#checking dataset information
df.info()
Output:
<class 'pandas.core.frame.DataFrame'>
RangeIndex: 511 entries, 0 to 510
Data columns (total 13 columns):
# Column Non-Null Count Dtype
--- ------ -------------- -----
0 CRIM 511 non-null float64
1 ZN 511 non-null float64
2 INDUS 511 non-null float64
3 CHAS 511 non-null int64
4 NOX 511 non-null float64
5 RM 511 non-null float64
6 AGE 511 non-null float64
7 DIS 511 non-null float64
8 RAD 511 non-null int64
9 TAX 511 non-null int64
10 PTRATIO 511 non-null float64
11 LSTAT 511 non-null float64
12 MEDV 511 non-null float64
dtypes: float64(10), int64(3)
memory usage: 52.0 KB
Summary Statistics
#checking summary of dataset
df.describe()
Output:
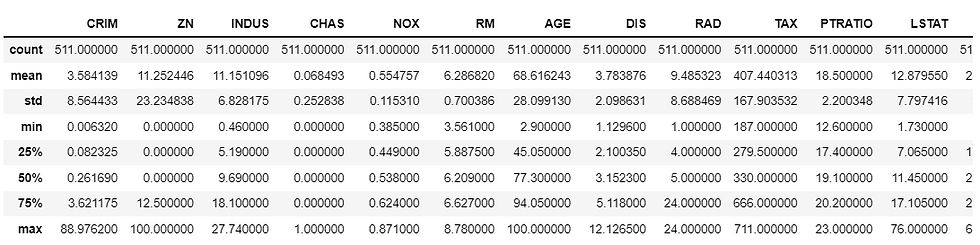
Feature Selection
#Deviding the target and features variables
X = df.drop('MEDV', axis = 1)
y = df['MEDV']
Normalize Features
from sklearn import preprocessing
min_max_scaler = preprocessing.MinMaxScaler()
X_scaled = min_max_scaler.fit_transform(X)
Split Dataset
#split
# Import 'train_test_split'
from sklearn.model_selection import cross_val_score
from sklearn.model_selection import train_test_split
# Shuffle and split the data into training and testing subsets
X_train, X_test, y_train, y_test = train_test_split(X_scaled, y, test_size=0.2, random_state=2)
# Success
print("Training and testing split was successful.")
K-fold Cross Validation
from sklearn.model_selection import cross_val_score
from sklearn.model_selection import KFold
import numpy as np
kf = KFold(n_splits=10)
SVR(Support Vector Regression)
from sklearn.svm import SVR
svr_rbf = SVR(kernel='rbf', C=1e3, gamma=0.1)
svr_rbf.fit( X_train, y_train)
scores = cross_val_score(svr_rbf, X_train, y_train, cv=kf)
print("Accuracy: %0.2f (+/- %0.2f)" % (scores.mean(), scores.std()))
Output:
Accuracy: 0.77 (+/- 0.12)
Decision Tree Regressor
from sklearn.tree import DecisionTreeRegressor
desc_tr = DecisionTreeRegressor(max_depth=3)
desc_tr.fit(X_train, y_train)
scores = cross_val_score(desc_tr, X_train, y_train, cv=kf)
print("Accuracy: %0.2f (+/- %0.2f)" % (scores.mean(), scores.std()))
Output:
Accuracy: 0.62 (+/- 0.12)
Draw the Graph
!pip install graphviz
!pip install pydotplus
import six
import sys
sys.modules['sklearn.externals.six'] = six
#draw tree
from sklearn.externals.six import StringIO
from IPython.display import Image
from sklearn.tree import export_graphviz
import pydotplus
feature_cols = ['CRIM', 'ZN', 'INDUS', 'CHAS', 'NOX', 'RM', 'AGE', 'DIS', 'RAD', 'TAX', 'PTRATIO', 'LSTAT']
dot_data = StringIO()
export_graphviz(desc_tr, out_file=dot_data,
filled=True, rounded=True,
special_characters=True, feature_names = feature_cols,class_names=['0','1'])
graph = pydotplus.graph_from_dot_data(dot_data.getvalue())
graph.write_png('boston.png')
Image(graph.create_png())
Output:
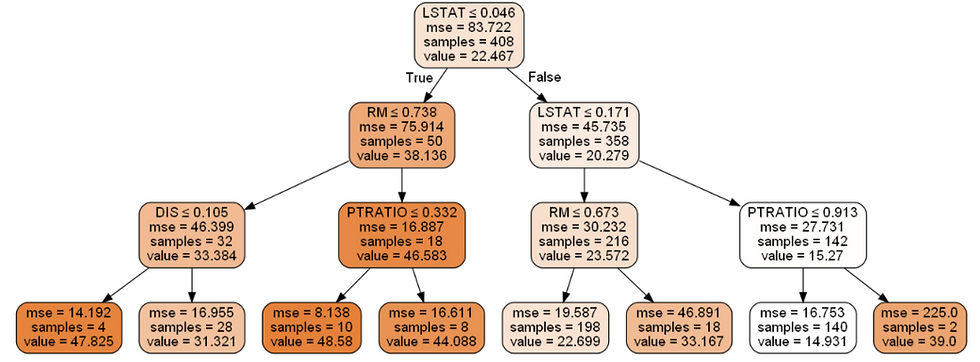
KNN
from sklearn.neighbors import KNeighborsRegressor
knn = KNeighborsRegressor(n_neighbors=3)
knn.fit( X_train, y_train)
scores = cross_val_score(knn, X_train, y_train, cv=kf)
print("KNN Accuracy: %0.2f (+/- %0.2f)" % (scores.mean(), scores.std()))
Output:
KNN Accuracy: 0.64 (+/- 0.12
Comments