Read Data From Drive
## import libraries
import pandas as pd
import numpy as np
location = '/content/drive/My Drive/..../Chicago_Crimes.csv'
from google.colab import drive
drive.mount('/content/drive')
# file Location
file_loc =location
# Read file
df = pd.read_csv(file_loc,header=0, sep=',', quotechar='"')
df.head()
Output:
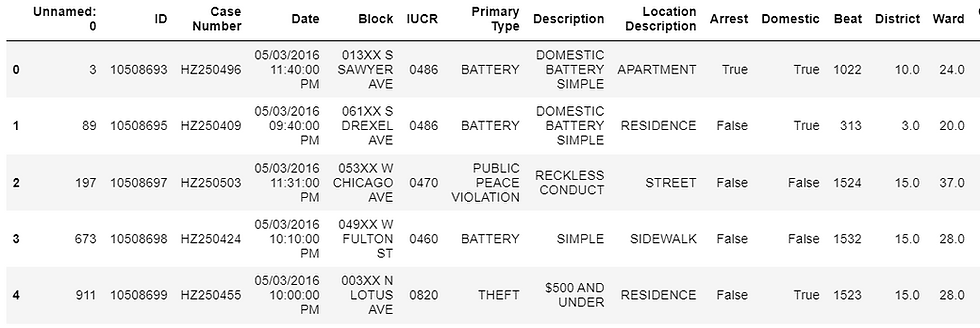
cols = df.select_dtypes([np.number]).columns
cols
Output:
Index(['Unnamed: 0', 'ID', 'Beat', 'District', 'Ward', 'Community Area',
'X Coordinate', 'Y Coordinate', 'Year', 'Latitude', 'Longitude'],
dtype='object')
#Select the "Arrest" feature
features = df[[i for i in cols]]
features['Arrest'] = df['Arrest']
features.dropna(inplace=True)
features.head()
Output:


Apply Label Encoder To Change String Into Integer
from sklearn.preprocessing import LabelEncoder
X = features.drop(['Arrest'],axis=1)
y = features['Arrest']
le = LabelEncoder()
y = le.fit_transform(y)
X.head()
Output:
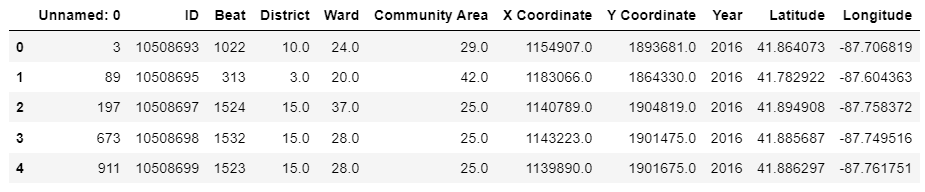
Split Dataset
# Splitting the dataset into the Training set and Test set
from sklearn.model_selection import train_test_split#.asarray(x).astype('float32')
X_train, X_test, y_train, y_test = train_test_split(np.array(X).astype('float32'), y.astype('float32'), test_size = 0.2, random_state = 42)
Apply Model
# Importing the Keras libraries and packages
from keras.models import Sequential
from keras.layers import Dense
# Initialising
classifier = Sequential()
# Adding the input layer and the first hidden layer
classifier.add(Dense(units=100,activation="relu", input_dim=X_train.shape[1]))
#classifier.add_weight(shape=(5,72))
# Adding the second hidden layer
classifier.add(Dense(250, activation = 'relu'))
# Adding the third hidden layer
classifier.add(Dense(150, activation = 'relu'))
classifier.add(Dense(50, activation = 'relu'))
# Adding the output layer
classifier.add(Dense(units = 1, activation = 'sigmoid'))
# Compiling the delta bar delta algorithim
classifier.compile(optimizer = 'adadelta',
loss = 'mse',
metrics = ['accuracy'])
classifier.fit(X_train, y_train, batch_size = 100, epochs= 5)
Output:
Epoch 1/5 11357/11357 [==============================] - 28s 2ms/step - loss: 0.2620 - accuracy: 0.7380 Epoch 2/5 11357/11357 [==============================] - 25s 2ms/step - loss: 0.2620 - accuracy: 0.7380 Epoch 3/5 11357/11357 [==============================] - 25s 2ms/step - loss: 0.2619 - accuracy: 0.7381 Epoch 4/5 11357/11357 [==============================] - 25s 2ms/step - loss: 0.2615 - accuracy: 0.7385 Epoch 5/5 11357/11357 [==============================] - 24s 2ms/step - loss: 0.2619 - accuracy: 0.7381
<tensorflow.python.keras.callbacks.History at 0x7fa9281a8150>
Evaluate Model
## evaluating the model
print(classifier.evaluate(X_test,y_test))
Output:
8873/8873 [==============================] - 13s 1ms/step - loss: 0.2600 - accuracy: 0.7400
[0.2599650025367737, 0.7400349974632263]
# Initialising the ANN
ann = Sequential()
# Adding the input layer and the first hidden layer
ann.add(Dense(units=100, input_dim=X_train.shape[1]))
#classifier.add_weight(shape=(5,72))
# Adding the second hidden layer
ann.add(Dense(250, activation = 'relu'))
# Adding the third hidden layer
ann.add(Dense(150, activation = 'relu'))
ann.add(Dense(50, activation = 'relu'))
# Adding the output layer
ann.add(Dense(units = 1, activation = 'sigmoid'))
# Compiling the delta bar delta algorithim
ann.compile(optimizer = 'adam',
loss = 'mse',
metrics = ['accuracy'])
# Fitting the ANN to the Training set
ann.fit(X_train, y_train, batch_size = 100, epochs= 5)
Output:
Epoch 1/5 11357/11357 [==============================] - 25s 2ms/step - loss: 0.2617 - accuracy: 0.7383 Epoch 2/5 11357/11357 [==============================] - 25s 2ms/step - loss: 0.2622 - accuracy: 0.7378 Epoch 3/5 11357/11357 [==============================] - 25s 2ms/step - loss: 0.2616 - accuracy: 0.7384 Epoch 4/5 11357/11357 [==============================] - 25s 2ms/step - loss: 0.2617 - accuracy: 0.7383 Epoch 5/5 11357/11357 [==============================] - 25s 2ms/step - loss: 0.2616 - accuracy: 0.7384
<tensorflow.python.keras.callbacks.History at 0x7fa9aa43d210>
ann.evaluate(X_test,y_test)
Output:
8873/8873 [==============================] - 13s 1ms/step - loss: 0.2600 - accuracy: 0.7400
[0.2599650025367737, 0.7400349974632263]
a = np.array(X)
na = a.reshape(a.shape[0],11,-1)
na=na.reshape(-1,1)
na = na[:20000]
na =na.reshape(-1,20,1)
na.shape
Output:
(1000, 20, 1)
Bar-Delta-Bar
import numpy as np
import matplotlib
import matplotlib.pyplot as plt
num_examples = len(na)
input_dim = 20
meta_step_size = 0.05
h = np.zeros((input_dim, 1))
w = np.random.normal(0.0, 1.0, size=(input_dim, 1))
beta = np.ones((input_dim, 1)) * np.log(0.05)
alpha = np.exp(beta)
s = np.zeros((input_dim, 1))
target_num = 5
def generate_task():
for i in range(target_num):
s[i] = np.random.choice([-1, 1])
def set_target(x, examples_seen):
if examples_seen % 20 == 0:
s[np.random.choice(np.arange(target_num))] *= -1
for i in range(target_num, input_dim):
s[i] = np.random.random()
return np.dot(s.transpose(), x)[0, 0]
def main(X):
alpha_mat = np.zeros((num_examples, 2))
global h, w, beta, alpha
generate_task()
for example_i,x in enumerate(X):
y = set_target(x, example_i + 1)
estimate = np.dot(w.transpose(), x)[0, 0]
delta = y - estimate
beta += (meta_step_size * delta * x * h)
alpha = np.exp(beta)
w += delta * np.multiply(alpha, x)
h = h * np.maximum((1.0 - alpha * (x ** 2)), np.zeros((input_dim, 1))) + (alpha * delta * x)
alpha_mat[example_i, 0] = alpha[0] # relevant feature
alpha_mat[example_i, 1] = alpha[19] # irrelevant feature
x_length = np.arange(1, num_examples + 1)
plt.errorbar(x_length, alpha_mat[:, 0].flatten(), label='relevant feature')
plt.errorbar(x_length, alpha_mat[:, 1].flatten(), label='irrelevant feature')
plt.legend(loc='best')
plt.show()
plt.savefig('/content/drive/My Drive/..../DeltaBarDelta.png')
main(na)
Thanks! If you need any help related to advance machine learning then Contact Us or send your requirement details directly at
realcode4you@gmail.com
Comments